Lire ce livre blanc en Français
5 reasons why you need Analytics Governance now
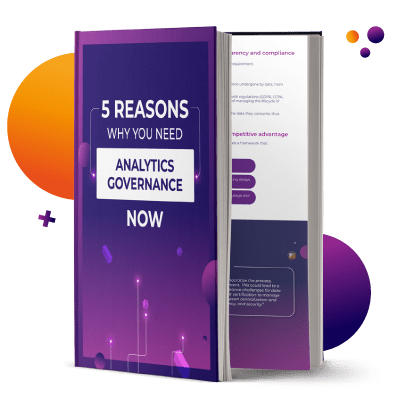
Introduction
In a world where data has become the backbone of decision-making, Analytics Governance ensures that business insights remain accurate, consistent, and reliable. While traditional Data Governance focuses on data integrity, quality, and security, Analytics Governance complements it by addressing the “last mile”: validating, certifying, and managing analytical content such as dashboards and decision-making reports. Together, they guarantee accurate, consistent, and trustworthy business insights.
Without proper governance at this stage, even high-quality data can lead to misleading conclusions, financial losses, or compliance risks. Ensuring the reliability of insights is no longer optional, it is a strategic necessity.
At Wiiisdom, we believe in a future where organizations can confidently leverage their data. This white paper highlights why Analytics Governance has become essential in 2025 through five key reasons and provides concrete solutions to fully capitalize on this approach.
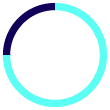
75.9% of organizations have BI governance policies,
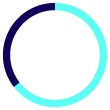
Yet 66% of errors in dashboards have led to negative consequences,
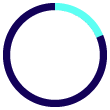
Quality control in BI is a top investment priority, but only 20% of organizations perform continuous validations.
This paradox highlights the gap between recognition and maturity in Analytics Governance. Many companies still rely on basic processes, far from advanced practices like automated certification or continuous monitoring.
To bridge this gap, this white paper will:
- Identify the five key reasons why Analytics Governance is essential in 2025 and discover how it enhances data management and decision-making,
- Anticipate challenges linked to AI adoption and regulatory changes,
- Provide actionable strategies to maximize data value while minimizing risks.
Whether you are a data or analytics leader, a data professional, or a strategic decision-maker, this guide will help you strengthen trust in your analytics.
“Let’s explore what 2025 holds
for Analytics Governance.”
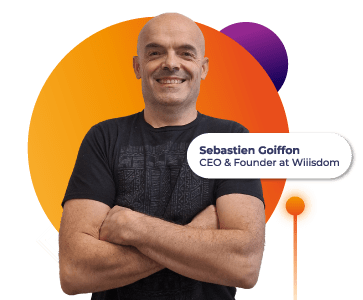
Part 1: Reason 1 – AI at the heart of the analytical revolution – essential governance in a changing environment
Redefining data management in the age of Artificial Intelligence (AI)
Artificial Intelligence (AI) is revolutionizing traditional data management approaches. Analytical tools, once reserved for a technical elite, are becoming accessible to all. This democratization leads to an exponential production of analytical content but also brings major challenges: inconsistent data, decision-making biases, and increasing complexity of analytical environments.
Anticipating risks associated with the massive production of analytical content
The widespread adoption of artificial intelligence and the democratization of analytics tools have led to an explosion in the production of analytical content within companies. While the rise of AI enables faster and more autonomous decision-making, it also brings significant challenges that organizations must anticipate and address to fully maximize its benefits.
1. Explosion of data volumes: A challenge for quality control
The exponential growth of data volumes, combined with the widespread digitalization of business functions, has led to a surge in use cases and a diversification of user profiles. As more non-experts handle analytics tools, the risk of misinterpretation, improper use, or reliance on inaccurate data increases significantly.
This reinforces the need for strong data quality controls and governance mechanisms to prevent errors from influencing critical business decisions. This evolution also brings several challenges:
- Information overload: Teams are drowning in an abundance of often redundant or contradictory reports, complicating decision-making and diminishing user trust and usage. 66% of errors identified in dashboards lead to negative consequences such as financial losses or reputational damage, underscoring the need to strengthen quality control processes,
- Compromised quality: The proliferation of content makes it difficult to identify, prevent, and correct errors or inconsistencies,
- Lack of prioritization: Critical data can be drowned in a flood of secondary analyses, thereby reducing their strategic impact.
Which solutions?
- Set up a centralized governance system to monitor and validate analytical content produced on a large scale and in an automated fashion,
- Use algorithms to automatically identify duplicates or obsolete reports. Cataloging or labeling content is another way of identifying trusted content,
- Provide targeted training and clear guidelines for non-data specialists to improve their ability to interpret and use data correctly,
- Implement role-based access and approval workflows to ensure that key insights are reviewed before being widely distributed.
2. Increasing errors: An exponential impact on strategic decisions
Even small errors in data or analytics can have disproportionate consequences when they propagate into decision-making or operational processes. These errors can result from:
- Misinterpretation: Non-expert users may draw erroneous conclusions based on incorrect or misrepresented data,
- Technical problems: Errors in calculations, integrations, or underlying models can go unnoticed,
- Rapid propagation: One error in a dashboard can affect dozens, even hundreds, of downstream decisions.
- Unrestricted access to sensitive data: Without clear governance rules, users may access and manipulate data beyond their expertise, increasing the risk of errors and misinterpretations.
Which solutions?
To mitigate these risks, organizations should:
- Automate upstream data verification processes to reduce human error,
- Train users to recognize and report anomalies, fostering a culture of data accountability,
- Implement automatic alert systems to quickly identify inconsistencies,
- Implement role-based access controls to ensure that users can only interact with data relevant to their role, balancing data democratization with governance.
These measures require strong governance policies that align with the company’s broader security framework, ensuring both data accessibility and integrity.
3. Fragmentation of standards: An obstacle to harmonization
In large companies, teams and departments often work with their own methods and analytical tools. This leads to a fragmentation of governance standards, with important consequences:
- Lack of uniformity: KPI definitions vary across teams, complicating comparisons and strategic alignments,
- Collaboration issues: Analytical silos hinder the sharing of relevant information between departments,
- Time loss: Teams spend considerable time interpreting or consolidating data from disparate sources.
Which solutions?
- Establish clear standards for the collection, storage, and analysis of data across the organization,
- Encourage the use of a centralized platform that enforces uniform governance rules,
- Involve a dedicated analytics governance officer to ensure consistent application of these standards.
Automation and intelligence: The pillars of tomorrow’s governance
Automation and artificial intelligence are no longer just about simplifying repetitive tasks; they have become strategic levers for establishing proactive and robust analytics governance. These technologies allow companies to manage massive volumes of data with increased efficiency while reducing human errors. In 2025, these tools will be essential for ensuring reliable analyses and informed decision-making. Here is how these pillars manifest in Analytics Governance:
1. Data certification: Ensuring quality upfront
Automated data certification involves validating the accuracy of data before it is used in analyses or reports. This step is essential to avoid decisions based on erroneous information. Key benefits include:
- Systematic checks: Validation of data sets using defined criteria (consistency, completeness, accuracy),
- Reduced lead times: An automated process can certify large volumes of data in just a few minutes, compared with several days for manual verification,
- Standardization: Ensure uniform certification processes throughout the company.
2. Real-time anomaly identification: Detect problems before they impact users
AI excels in quickly analyzing large data sets to detect anomalies, even subtle ones. Modern tools enable:
- Continuous Monitoring: Learning algorithms detect deviations from expected behaviors, such as an unusual increase in financial indicators or errors in generated reports,
- Instant Alerts: Anomalies are reported in real-time, allowing teams to correct them before they lead to costly errors,
- Risk Prediction: Using historical analysis, AI anticipates potential problems and recommends preventive actions.
3. Traceability of analytical flows: Enhancing transparency and compliance
With the proliferation of data and regulations, traceability has become a major requirement. Automation enables:
- Data flow mapping: Clearly identify the origin, journey, and transformations undergone by data, from initial storage to final reports,
- Facilitated auditing: Provide detailed logs to demonstrate compliance with regulations (GDPR, CCPA, GxP, SOX, etc.) or to quickly respond to inspections, particularly in terms of managing the lifecycle of decision-making content,
- Enhanced transparency: End users can view the source and quality of the data they consume, thus bolstering their trust.
4. Optimization of decision-making processes: A competitive advantage
By combining certification, anomaly detection, and traceability, companies create a framework that:
- Reduces uncertainties in strategic decisions,
- Accelerates decision-making cycles by eliminating delays associated with manual validations,
- Also fosters innovation by removing barriers to usage and thus to the adoption of new technologies.

Wiiisdom’s vision
“The integration of generative AI into analytics will democratize the process, transforming all users into consumers and producers of content. This could lead to a massive proliferation of new content and pose major governance challenges for data and analytics leaders. Organizations must prioritize content certification to manage this situation, while D&A leaders must find a balance between centralization and decentralization to maintain governance, consistency, and security.”
Part 2: Reason 2 – Accelerating a data-driven culture
A cultural revolution in businesses
The rise of data as a central driver of decision-making is transforming not only processes but also mindsets within organizations. As companies increasingly rely on trusted data to make decisions, it becomes essential for every employee to adopt a data-driven approach.
This transformation is based on two main pillars:
- Building trust in data: Decisions can only be meaningful if the data they are based on is reliable, verified, and contextualized,
- Aligning teams around shared goals: A data-driven culture is not solely about technology but a collective effort to transform data into a true competitive advantage.
Developing aligned skills and governance
The success of a data-driven culture requires a combination of technical and behavioral skills, as well as a robust governance framework to maximize its benefits.
1. Train “data-savvy” employees
To fully leverage data, it is crucial to move beyond skill silos and empower teams. This involves:
- Targeted training: Train not only data analysts but also business and operational teams to ensure they understand data fundamentals,
- Continuous education: Technology and methodologies evolve rapidly, requiring ongoing efforts to keep skills up to date,
- Developing a common language: To collaborate effectively, all stakeholders must share a common understanding of data and key KPIs.
2. Establishing collaborative governance processes
A data-driven culture relies on aligned and accessible governance. However, only 20% of organizations implement continuous validation of their analytical content, highlighting a gap between strategic ambitions and current operational practices.
- Clear definition of responsibilities: Roles must be well-defined, whether for data producers, users, or governance managers,
- Fostering a participatory approach: Decisions about data should involve a wide range of stakeholders to ensure their relevance and adoption,
- Centralization and transparency: Companies must establish standardized processes for collecting, validating, and sharing data while ensuring users trust its quality.
Discover real-life examples of companies that have built lasting trust in their analytics content through proactive governance, as shown in our case study with UCLA.
Towards a new organizational dynamic: The democratization of data
1. Making data accessible to all
For a data-driven culture to be effective, every employee must have access to relevant information and be able to interpret it:
- Intuitive platforms: Analytical tools must be easy to use, even for non-technical users,
- Contextualized insights: Providing raw data is not enough; it must be translated into actionable insights,
- Needs-based access: While respecting confidentiality constraints, it is essential that each user has access to the data required for their mission.
2. Measuring and celebrating data-driven success
Implementing a data-driven culture requires clear indicators of success to demonstrate the impact of this approach:
- Track data-driven performance: For example, shorter decision cycles, more accurate forecasts, or cost savings thanks to relevant analyses,
- Celebrating success: Highlighting successful projects and teams that make effective use of data encourages adoption and strengthens employee commitment.
The challenges of a data-driven culture
1. Overcoming resistance to change
Although the benefits are obvious, the transition to a data-centric culture can be perceived as an upheaval, or even a threat, by some employees. It is crucial to:
- Communicate the benefits: Show how data facilitates daily tasks and improves results,
- Support teams: Provide training and ongoing support to reduce fears linked to technological complexity.
2. Avoiding data overload
As data production accelerates, the risk of information overload increases. Companies must:
- Prioritize critical information: Highlight the data most relevant to strategic decisions,
- Structure data consumption: Offer customized dashboards and clear visualizations to avoid confusion,
- Labeling content: Identify and certify key data to guarantee its quality and relevance, while facilitating its use by different user profiles.

Wiiisdom’s vision
“To foster a data-centric culture, it is essential to adopt analytical governance solutions that build trust and encourage the use of data. Organizations need to promote the adoption of trusted analytics, leading to improved decision-making and optimized operational performance.”
Part 3: Reason 3 – Reinforcement of compliance and confidentiality standards
An increase in regulations requiring strict governance
Regulatory requirements for data usage, management, and protection have become unavoidable, forcing companies to adopt strict and proactive governance. Whether through regulations such as the GDPR in Europe (or the AI Act and its forthcoming arrival), the CCPA in California, or sector-specific directives (e.g. HIPAA for healthcare or Basel III for finance or GxP for pharmaceuticals), compliance requirements are constantly growing.
This trend reflects two major imperatives:
- Protect the rights of individuals in the face of massive data exploitation, by guaranteeing confidentiality and control,
- Ensure corporate transparency and accountability in the management of critical information.
Challenges for companies
The increasing power of these regulations poses complex challenges for organizations:
- Multi-jurisdictions: Companies operating in several countries have to juggle with sometimes contradictory regulations,
- Increasing documentation requirements: Audits and inspections demand detailed traceability of every data-related process,
- Severe penalties: Non-compliance not only leads to high fines but also a loss of reputation that’s hard to make up for.
Investing in governance solutions to ensure compliance and risk reduction
To address these challenges, companies must adopt a proactive approach to compliance, based on advanced technological solutions and rigorous governance practices.
1. Automate compliance to avoid human error
Modern governance solutions integrate automation tools that:
- Control access and permissions to sensitive data to minimize the risk of data leakage,
- Automatically audit data flows to ensure traceability and compliance,
- Provide real-time alerts in the event of process deviations or rule violations.
2. Implement governance policies adapted to each sector
Each sector has specific compliance needs:
- Finance: Management of critical customer data, financial risk control (Basel III, SOX),
- Banking: Risk-critical data management to avoid systemic crises (BCBS-239),
- Pharma and healthcare: Patient data confidentiality (GxP, HIPAA, FDA 21 CFR Part 11),
- Retail and e-commerce: Protecting customer information and payments (PCI DSS, GDPR).
Companies need to develop internal policies that not only comply with industry regulations but also adapt to future developments.
3. Educating teams for effective governance
Technology alone is not enough; successful governance also relies on informed and involved teams. This includes:
- Ongoing awareness-raising: Regularly train employees on new regulations and their implications,
- Impose internal standards: Establish clear guidelines on data collection, use and destruction,
- Encouraging best practices: Rewarding initiatives that strengthen compliance and reduce risk.
The benefits of proactive governance
By investing in the right governance solutions, companies can turn compliance from a constraint into a strategic advantage:
- Reduce financial and legal risks: Minimize regulatory fines and litigation,
- Improved stakeholder confidence: Transparent data management inspires confidence in customers, investors and partners,
- Process optimization: Automated solutions reduce operational costs while increasing accuracy and efficiency.

Wiiisdom’s vision
“Organizations must establish strategic partnerships to address increasing regulatory constraints while improving operational efficiency and aligning their actions with corporate strategies to achieve ambitious outcomes. By adopting innovative solutions from the outset, they can quickly achieve tangible results at every stage of the data lifecycle—from analytical consumption to AI-generated insights. This approach fosters a culture of quality and informed decision-making while delivering validated analyses and insights more efficiently.”
Part 4: Reason 4 – Transitioning from a reactive world to a proactive world through automated certification of analytical content
From reactivity to proactivity thanks to analytical certification
In a world where decisions increasingly rely on analytical content, users continue to face challenges related to data quality, dashboard performance, or biased analyses. These issues not only undermine user trust but also slow down decision-making processes.
Automated and dynamic certification of analytical content represents a major shift: it transforms a reactive system—where problems are addressed after the fact – into a proactive system capable of identifying and resolving anomalies before they impact users. This change allows companies to ensure an optimized user experience and establish a lasting framework of trust.
Automated certification as a pillar of analytical reliability
Automated certification processes help establish high standards for data and analytical quality. Currently, only 45% of BI professionals consider quality testing for analytical content a priority, highlighting the need to raise awareness among organizations about its strategic importance. Specifically, this requires focusing on three main areas:
1. Guaranteed quality at every stage
- Automated certification solutions systematically validate reports and dashboards before and after publication, verifying criteria such as:
- Data accuracy: Consistency between sources and analyses,
- Technical performance: Speed of loading and fluidity of interaction,
- Calculation reliability: Detection of potential errors in formulas or aggregations.
2. Increased user confidence
- The visual certification of reports and their date (via badges or labels, for example) reassures users of the quality and reliability of the information they consume,
- By guaranteeing reliable analytic content, companies reduce the risk of erroneous decisions based on biased data, empower consumers, and foster trust.
3. Easier adoption of analytical tools
- When users know that their reports and analyses have been certified, they are more inclined to use the analytical tools at their disposal, thus avoiding reprocessing,
- Certification also unifies quality standards across the company, reducing disparities between departments.

Wiiisdom’s vision: Building a trusted analytical world
“Our vision: a world where everyone can freely and confidently make decisions based on reliable data. Certification provides a visual reassurance to users in their data consumption.”
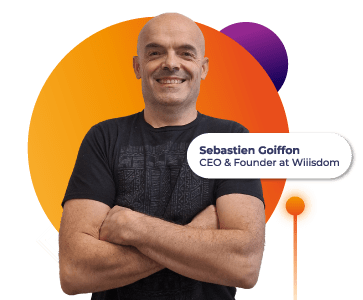
At Wiiisdom, this vision guides our commitment to providing solutions that build lasting trust in analytical content. Through automated certification, we help organizations guarantee data quality and reliability while simplifying user adoption.
Part 5: Reason 5 – Maximizing ROI through optimized data management processes
Reduce costs and maximize data value
Data management, while essential to powering analytical strategies, represents a significant proportion of companies’ operational costs. As data volumes continue to grow, so do these costs, presenting companies with a twofold imperative:
- Reduce the costs associated with data collection, storage, and analysis,
- Maximize the value extracted from data to improve performance and justify investments.
To achieve this balance, companies are increasingly turning to Analytics Governance solutions and automation tools, enabling them to streamline their processes while unleashing the strategic potential of their data.
The Importance of Effective Analytics Governance
Effective Analytics Governance plays a key role in optimizing data management processes, particularly by identifying and eliminating inefficiencies. Here are the main action levers:
1. Streamline data flows
- Data centralization: Consolidation of data from multiple sources to reduce redundancy and duplication,
- Standardization of processes: Implementation of uniform standards for data collection, storage and processing,
- Automate repetitive tasks: Reduce manual effort in analytical processes by integrating intelligent tools.
2. Improve data quality and reliability
- Proactive anomaly detection: Identify errors or inconsistencies before they affect analyses,
- Continuous monitoring: Implementation of mechanisms to ensure that data remains compliant with established quality standards,
- Content lifecycle management: Implementation of controls and validation for the delivery, modification, and validation of analytical content,
- Dynamic and automatic content certification: Adding an extra layer of validation to ensure that data is ready for use.
3. Reduce operational lead times
- By optimizing data flows, companies can reduce the time needed to produce usable analyses,
- Streamlined processes also enable faster response to internal and external demands, increasing the organization’s overall responsiveness.
How can a data-driven culture scale in a company if trust is not established?
Above all, a data-driven culture is based on trust. If internal and external users do not perceive data as reliable, analytical initiatives are likely to fail, whatever the technologies employed.
To effectively scale a data culture, it is essential to:
- Establish clear validation mechanisms: Automated certification processes must be integrated to reassure users about data quality,
- Promote transparency: Users need to understand the origin, processing, and limitations of the data they utilize,
- Provide intuitive and reliable tools: Ease of use and the performance of analytical tools strengthen user engagement.
In the absence of trust, organizational silos persist, and data-driven decisions are called into question, compromising the overall efficiency of analytical processes.
Optimization of data management processes: what are the benefits?
By optimizing data management processes, companies can:
1. Maximize analytical ROI
Optimized data management enables resources to be focused on high-value-added activities. By reducing inefficiencies, companies can derive more relevant insights and improve their competitiveness.
Moreover, by reinforcing data governance policies from the earliest stages of the value chain, they ensure better data quality and more strategic use.
2. Reduce operational costs
Automation and standardization reduce the costs associated with manual tasks or disparate systems. A streamlined infrastructure also reduces expenditure on unnecessary storage or duplication of data, while limiting service interruptions and the number of tickets sent to BI teams.
In addition, the adoption of innovations such as GenAI analytics contributes to significant operational gains, boosting overall performance.
3. Enhancing organizational agility
Teams can access critical information more quickly, enabling them to respond to market developments with greater responsiveness. Effective governance also reduces friction between departments, fostering fluid collaboration.
At the same time, the scaling of analytic uses supports a data-driven transformation, enabling a better amortization of analytics investments while minimizing risks (reputational, regulatory, business,…)
Optimizing data management processes isn’t just about reducing costs: it’s a strategic opportunity to maximize the value extracted from data and establish a robust, scalable analytics culture. Companies that invest in effective governance solutions will be better positioned to meet tomorrow’s challenges while ensuring a tangible return on investment.
Conclusion
In 2025, Analytics Governance is a critical strategic lever for ensuring decisions are based on reliable and secure data. The white paper highlights AI, data-driven culture, enhanced compliance, automated certification, and process optimization as key drivers of this transformation.
Yet, the full value of these investments, people, processes, and technology, depends on last-mile governance. If data fails to inspire trust at the point of use, the entire system is at risk. Ensuring last-mile reliability not only protects these investments but also accelerates decision-making by delivering accurate data to the right people, at the right time.
Success in this new landscape requires resilient Analytics Governance tailored to your needs. Now is the time to strengthen last-mile data integrity, optimize processes, and build trust across your organization.